AI: The present and future of immersive training
Limitations of traditional training
There are several common limitations in traditional training; let's take a look at them below.
Unidirectional approach and student passivity
Traditional training tends to focus on the instructor, who conveys knowledge in a one-way manner through lectures, readings, and practical exercises. In this model, students adopt a passive role as information receivers, limiting their
engagement and participation, which hinders the effective construction of learning.
Lack of personalization and adaptation
One of the primary challenges of traditional training is its inability to personalize content and teaching methodologies. Programs are often rigid, hindering customization to individual needs, interests, and learning paces. This can lead to demotivation and disengagement, especially in virtual environments where completion rates are significantly lower.
Difficulty in evaluating practical skills at scale
Assessment in traditional training primarily relies on exams and standardized tests, making it challenging to effectively evaluate practical skills, particularly in large groups. This limitation affects both technical competencies and soft skills, which require more dynamic and personalized approaches.
Limitations in Soft and Hard skills training
Addressing both technical (hard) and interpersonal (soft) skills in an agile and large-scale manner is a challenge in traditional models. The lack of resources to offer practical, up-to-date, and contextualized training experiences limits the effectiveness of learning and preparedness for ever-changing work environments.
Unrealistic and expensive simulations
Traditional simulations for skill development are often costly, difficult to scale, and, in many cases, unable to safely and economically replicate critical or infrequent situations. This restricts students' ability to face real and complex scenarios in a controlled environment.
A gateway to a world of possibilities
We are at a moment where generative artificial intelligence is transforming content creation by overcoming many of the previous limitations and shortcomings in this field. This technology enables the dynamic generation of materials in various formats—such as text, video, or multilingual virtual avatars—using internal knowledge bases, adapting content based on the specific needs of the user or the context.
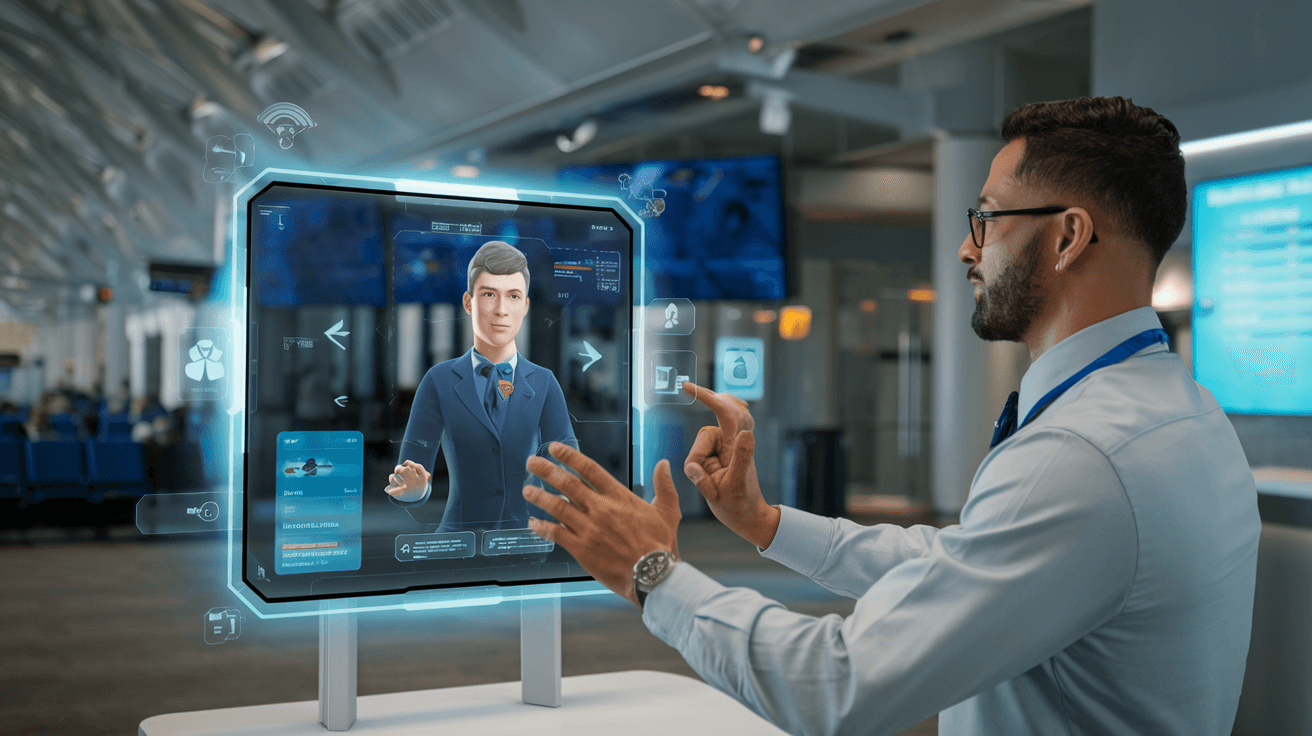
For example, in the educational and training environment, AI allows the creation of audiovisual presentations in which virtual avatars explain technical or procedural concepts, facilitating comprehension and access to information in multiple languages. These solutions enrich the learning experience, make knowledge
more accessible, and enable personalization that was previously difficult to achieve. Furthermore, generative AI automates repetitive tasks and accelerates content production, freeing up time for creativity and innovation in the design of training experiences.
In the immersive realm, AI will soon enable the creation of more realistic and personalized scenarios, where both the environment and virtual characters can respond in real-time to the user's actions. This evolution will make it possible to develop more interactive and individually tailored training experiences, which is expected to improve content quality and increase engagement and knowledge retention.
Generative AI opens the door to the development of many capabilities, which we can explore further.
The role of simulators in training development
One of these capabilities is AI-based simulators, which represent a distinct competitive advantage. For example, in areas like customer service and sales, they allow professionals to train soft skills through role-playing in realistic virtual simulations, such as managing difficult customers.
The design of these simulators has been optimized for ease of use: all that is needed is to define the context, objectives, the role of the AI, the tone of the conversation, the scenario, and the desired flow (e.g., greeting, security questions, problem analysis, cross-selling, and farewell), along with examples of good and bad practices. With this information, the model generates multiple scenarios adapted to different profiles. The scenarios can include real-life situations in highly immersive environments. Additionally, virtual assistants and intelligent tutors guide the user according to their progress, ensuring proper assimilation of content and facilitating the acquisition of the skills necessary to handle professional performance situations.
As examples of these applications in the aviation sector, a cabin crew member can be accompanied by a virtual tutor during an emergency drill on board, receiving real-time guidance and tips on safety protocols. Meanwhile, ground assistance staff can receive personalized instructions while simulating passenger boarding or baggage handling under changing operational conditions.
Generative AI enhances knowledge retention by providing contextualized and interactive training experiences. Unlike in-person role-playing, these simulations are scalable and allow for objective performance evaluation. Additionally, the system learns from the feedback it receives, continuously enhancing its precision and realism.
The data generated enables precise, sustained evaluation over time, feeding predictive models capable of anticipating training needs, detecting knowledge gaps, and recommending content tailored to each profile.
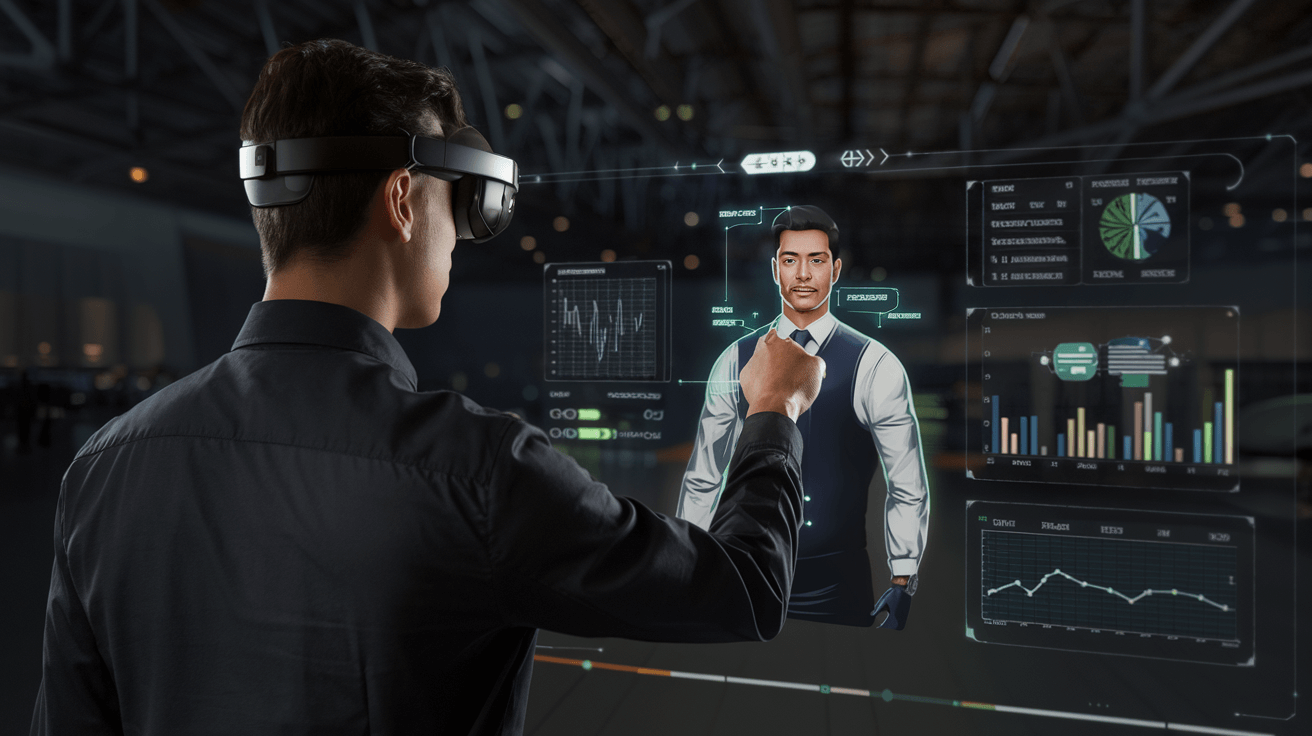
And that's not all: AI allows for the analysis of individual performance through machine learning models and the creation of scenarios based on personalized conversational flows, including emotional tone, key steps, and pedagogical objectives. The incorporation of Smart NPCs (intelligent non-playable characters) enriches the simulation by representing multiple profiles and responding coherently to the context, adding an extra level of realism and adaptability.
However, all these technological advancements are not without challenges, especially when it comes to their effective integration and acceptance within organizations.
Challenges in the use of Generative AI
Despite the growing interest and advancements in generative AI, significant barriers and reluctance to its adoption by businesses persist. One of the most prominent challenges is the fear of replacing the human trainer, especially in sectors like education, where the figure of the teacher is considered indispensable in many aspects.
This concern is further amplified by the lack of familiarity with the technology, leading some professionals to perceive AI as a threat or even an "enemy" that jeopardizes their traditional role.
Additionally, the integration of generative AI requires flexible technological infrastructures. It is essential to invest in open and model-agnostic architectures, allowing organizations to evolve and adapt to rapid technological changes without being tied to a single vendor or platform. This approach facilitates interoperability, scalability, and the ability to incorporate future innovations without major technical limitations.
Another crucial challenge is the concern about data privacy and security. Many companies fear that sensitive organizational information could be used to train external models or, worse, that confidential data could be leaked. This risk becomes a significant barrier to adopting generative AI-based solutions, especially in regulated sectors or those handling critical information.
Lastly, it is important to emphasize the need for training and awareness within organizations. To overcome these barriers, it is crucial to invest in team training and in creating a digital culture that promotes the responsible and ethical use of artificial intelligence. Only in this way can we fully harness the potential of generative AI, minimizing its risks while maximizing its benefits.
Future impact: How these technologies can transform training
The future of professional training is poised to be deeply transformed by generative AI. As illustrated, the trend points toward AI-driven learning environments, where traditional learning management systems (LMS) evolve into intelligent platforms. These new systems will integrate virtual tutors capable of customizing learning paths, monitoring each user's progress, and proactively anticipating training needs, dynamically adapting to individual goals and paces.
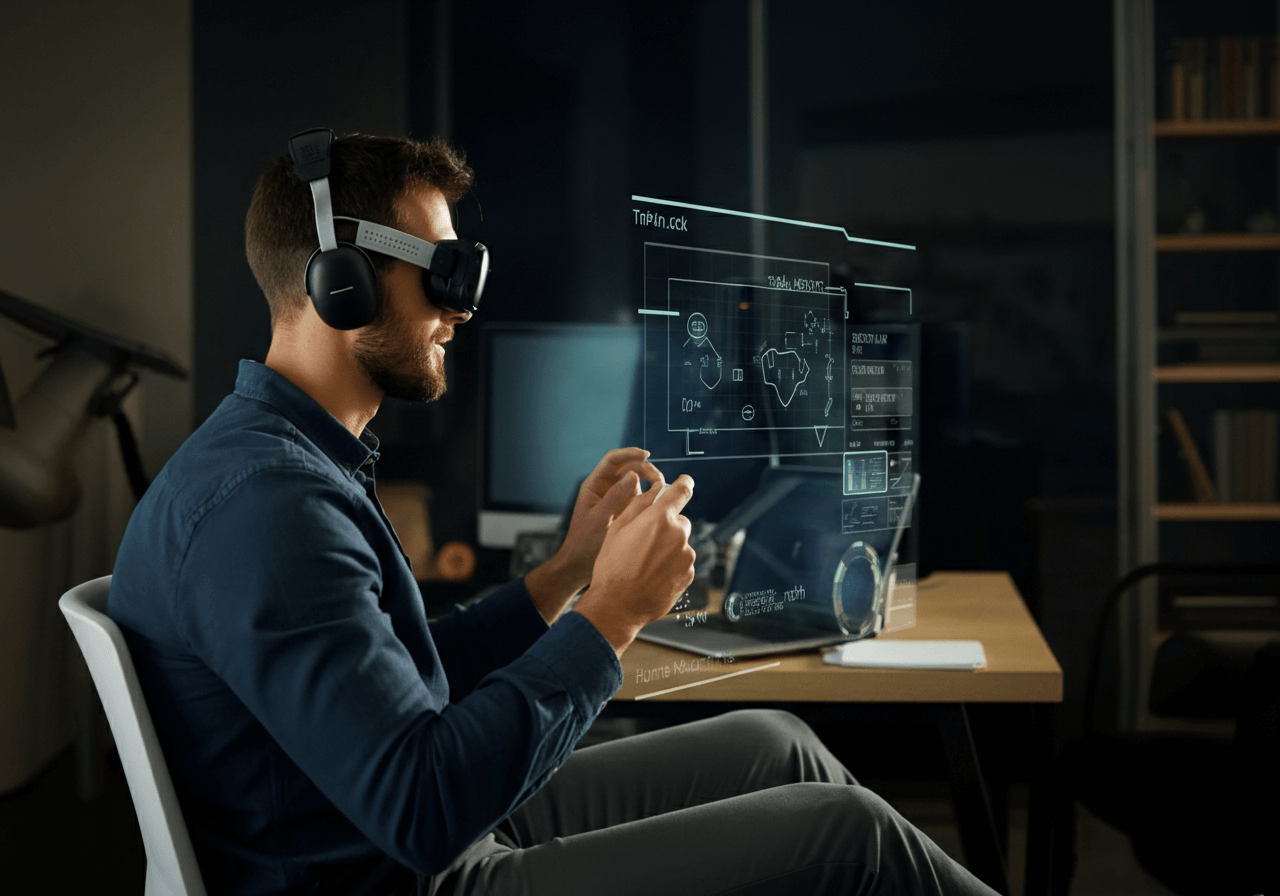
In the medium term, it is expected that training will be organized around multi- agent systems, where different AI models, each specialized in a specific area, collaborate to offer a much more precise, contextualized, and efficient learning experience. For example, one agent specialized in verbal communication may interact with the user, while another transforms that interaction into written communication, and a third ensures that the content aligns with corporate policies. This collaboration among agents will enable much more sophisticated personalization and oversight than current systems allow.
Moreover, generative AI will enable the simulation of highly realistic training scenarios, recreating nearly any potential future situation. This will be especially valuable in sectors such as aviation, where personnel will be able to face critical challenges and emergency situations in safe virtual environments, without any risks for real safety. These advanced simulations will facilitate the development of both technical and emotional skills, enhancing preparation and the ability to respond to complex situations.
Other technologies complementing AI in immersive training experiences
Immersive training experiences driven by artificial intelligence are significantly enhanced when integrated with other emerging technologies. Virtual reality (VR) and augmented reality (AR) play a crucial role in creating more realistic, interactive, and dynamic learning environments. While VR enables total immersion in digital scenarios, AR adds layers of information to the real world, aiding the understanding of complex concepts and allowing interaction with virtual
objects within the physical environment. In some cases, mixed reality (MR) combines both approaches, achieving seamless integration between the physical and digital worlds, ideal for collaborative simulations and advanced educational contexts.
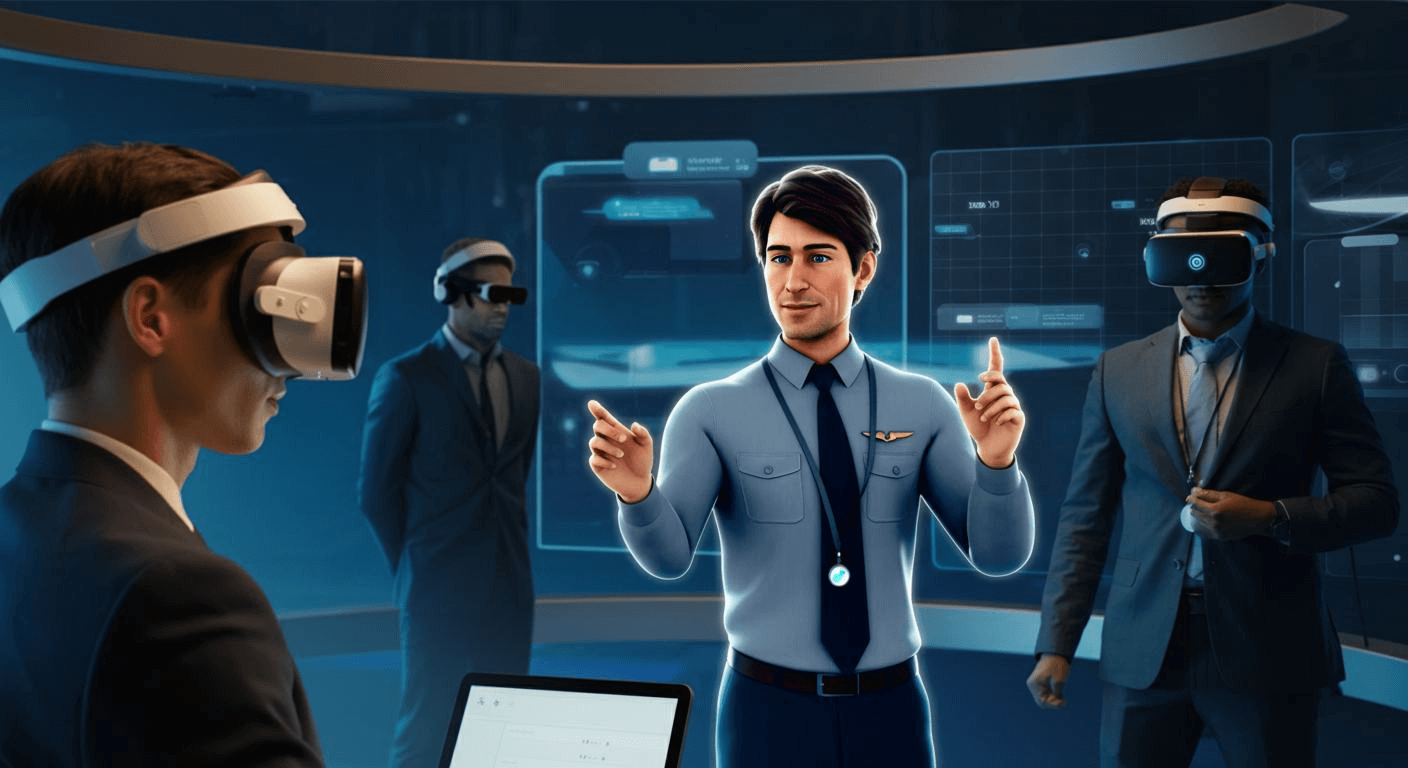
Advanced analytics and reporting systems, powered by artificial intelligence, offer the ability to personalize reports and deeply measure the impact of training experiences. With these tools, businesses can gather valuable data to continuously adjust and improve their training programs. Predictive analytics also allows for anticipating training needs and identifying areas for improvement, optimizing educational decision-making.
Machine learning algorithms focused on the adaptive learning paradigm complement this ecosystem by enhancing the personalization of learning. These systems can adapt content and activities in real-time based on each user’s performance and preferences, identifying behavioural patterns, anticipating difficulties, and recommending personalized learning paths to maximize training effectiveness.
Finally, voice interfaces and natural interactions, such as gesture and facial expression recognition, facilitate navigation and task execution within simulated environments. These technologies improve the accessibility and efficiency of training platforms, providing a more intuitive, seamless, and engaging experience for all types of users.
Together, the convergence of these technologies creates a training ecosystem where AI, alongside immersive solutions, analytics, and advanced interfaces, ensures richer, safer, more personalized, and accessible learning experiences.
Final conclusions
The strategic application of generative AI has the potential to overcome many of the current limitations facing training in the aviation sector, providing more effective, motivating learning experiences aligned with the challenges of a rapidly evolving environment.
Thanks to AI’s ability to personalize content, anticipate needs, and simulate complex scenarios, training becomes not only more relevant but also more engaging and tailored to the real-world demands of aviation personnel.
In this new horizon, where traditional training coexists with the transformative power of AI, technology consolidates itself as a catalyst for efficiency and personalization. However, the true qualitative leap for aviation companies does
not solely rely on the sophistication of algorithms or technological innovation but on the organizations' ability to embrace a profound cultural transformation.
Strategically integrating generative AI requires an open, flexible, and change- oriented mindset, where collaboration between humans and machines is seen as an opportunity for growth rather than a threat.
The ultimate challenge, therefore, is both technological and human. Only by cultivating a receptive and adaptive organizational culture will it be possible to turn the promise of smarter, scalable, and immersive training into a tangible and transformative reality for airline companies and their professionals. Thus, the integration of AI in training will not only advance processes but also redefine the future of learning in the sector, preparing organizations to successfully face the challenges of tomorrow’s aviation.